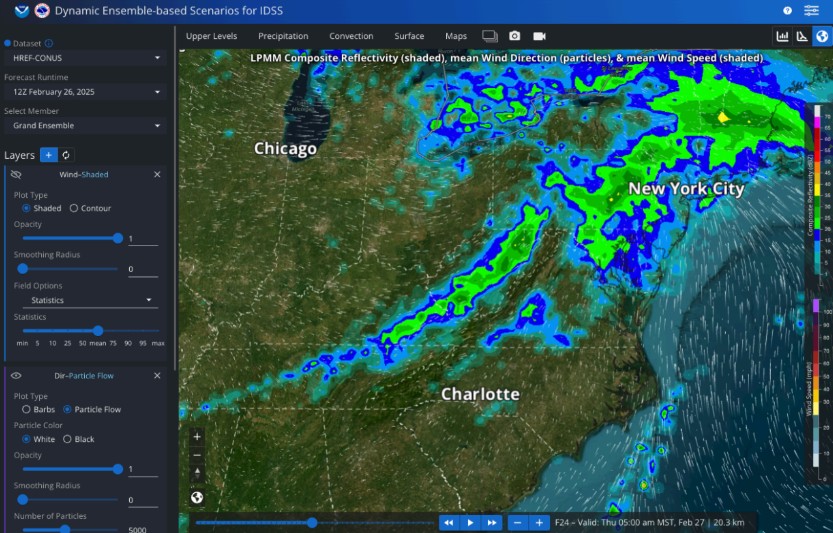
*This article was originally written February 27, 2025.
Social science researchers at the National Oceanic and Atmospheric Administration’s (NOAA) Global Systems Laboratory (GSL) have completed the first comprehensive evaluation of one of GSL’s flagship decision-support tools. The Dynamic Ensemble-based Scenarios for Impact-based Decision-Support Services (DESI) is a web-based application that allows weather forecasters to slice and dice ensemble weather model data to extract probabilistic information about weather forecasts. Need to know the probability of getting four inches of snow in six hours? DESI can tell you. What about the maximum possible rainfall totals for next Saturday? DESI knows.
But up until now, there was something that DESI did not know, at least not to the extent that was desired. DESI’s users, their decision spaces, how they use weather information from DESI to develop and communicate forecasts, and whether DESI was meeting their information and decision-support needs had not been methodically studied. GSL social scientists jumped into action in summer of 2024 to begin scoping the evaluation design by developing an interview guide. In all, GSL social scientists conducted 45 semi-structured interviews—primarily with National Weather Service (NWS) meteorologists. These interviews comprised over 40 hours of discussions about when, where, why and how DESI and probabilistic forecast information are used by forecasters.
Through this project, GSL’s social scientists completed a documented workflow of NWS meteorologists, informing a broader understanding of their operational contexts that can be applied and built upon in future research studies and evaluations. They identified new ways to provide DESI training to forecasters and examined how DESI influences some of the hardest decisions that these forecasters have to make. The researchers learned how DESI informs meteorologists’ communication of the forecast to their partners and the public while also documenting future development ideas that are consistent with the vision and needs of our end users.
Among other findings, DESI increased the self-reported confidence of forecasters by providing an efficient and centralized platform that integrates seamlessly into forecaster workflows. This helps reduce their mental load and stress—components which participants described as a bane to their confidence.
The probability of a weather variable meeting a specific threshold-–often called the probability of exceedance–was the most commonly used type of probabilistic information for data interrogation and partner communication among interviewed meteorologists when compared to use of percentiles and ranges. Users emphasized the need for even greater development of DESI’s communication, graphic creation, and decision-support capabilities to enable them to continue meeting the growing demand for their partners’ decision-support needs.
Users expressed excitement and a strong sense of optimism for the future of DESI. One study participant summed up their feelings toward DESI, saying, “It is the most powerful up-and-coming tool I think that I’m using right now, and I absolutely love it.” Another echoed this revolutionary tone, adding, “You’re moving into the future.” This work by GSL’s social scientists will directly inform upcoming releases, ensuring DESI continues to evolve and meet the growing needs of its users.